What is ML?
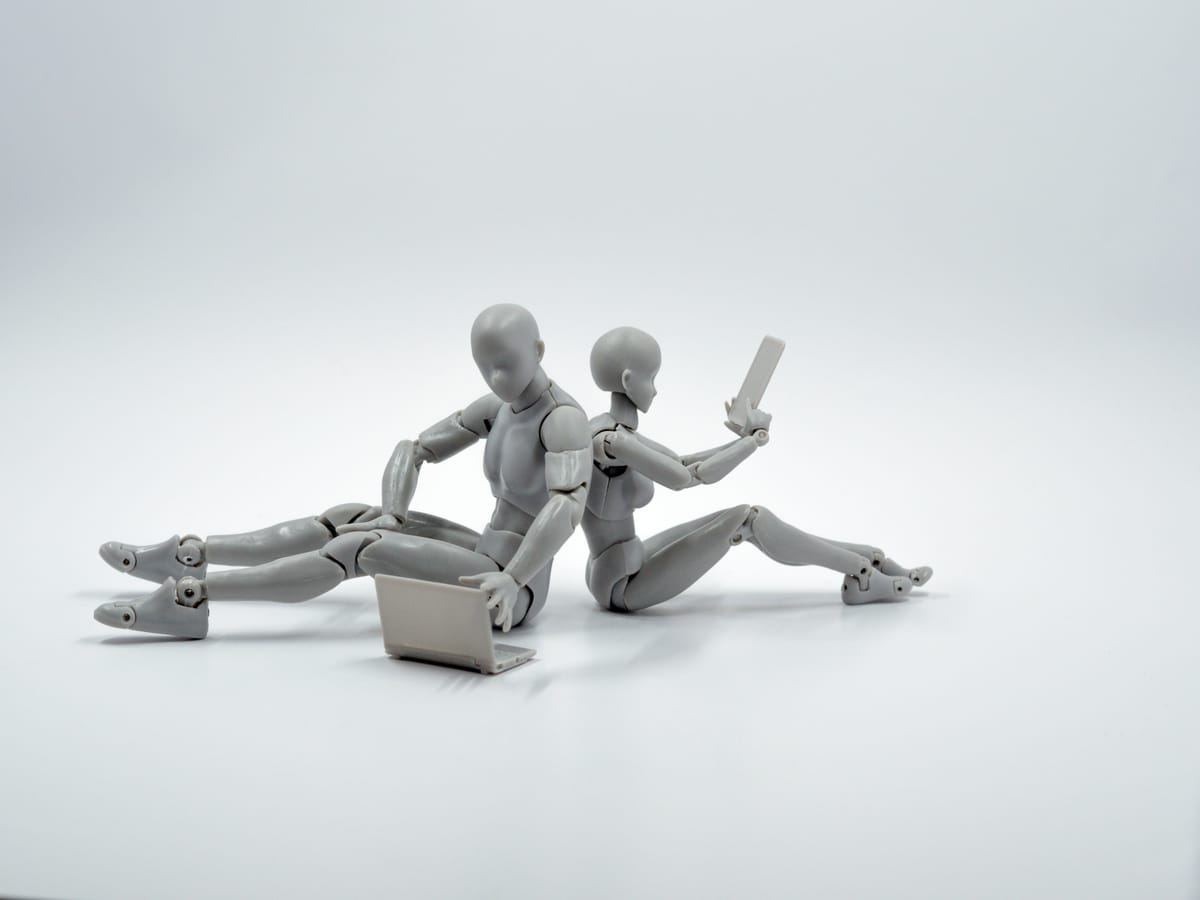
Machine learning is everywhere these days. Mostly in newspapers, but it's seeping into many real life, actual use cases. But what is it actually?
If you read only articles on TechCrunch, Forbes, Business Insider or even MIT Technology Review, you'd think it's something that brings Model T800 to life soon, or that it will cure cancer and make radiologists useless, or that it will enable humans to upload their minds to the cloud and live forever, or that it will bring fully self driving cars by the end of the year (every year for the last 5 years).
Many companies want to get in on the ML bandwagon. It's understandable: 1) that's where the money is (some 10 billion dollars were invested in it in 2018) and 2) correctly done, applied to the right problems, ML can actually be really valuable, either by automating things that were previously done with manual labor or even by enabling things that were previously unfeasible.
But at the same time, a lot of ML projects make unrealistic promises, eat a lot of money and then deliver something that doesn't work well enough to have a positive ROI. The ML engineers and researchers are happy, they got payed, analyzed the data and played around with building ML models, and maybe even published a paper or two. But the business is not happy, because they are not better off in any way.
This is not a new phenomenon. Artificial Intelligence, of which Machine Learning is a subdomain of, has been plagued by similar bubbles ever since it was founded. AI has gone through several AI winters already, in the 60s, 80s and late 90s. Big promises, few results.
To paraphrase Battlestar Galactica, "All this has happened before, all this will happen again but this time it's different". But why is it different? More about that tomorrow.